The notion of instantaneous, error-free translation between diverse languages has long been a staple of science fiction. Perhaps the most famous example is the Universal Translator seen in the television series Star Trek. Another less practical example comes from Douglas Adams’s humorous 1978 BBC Radio 4 programme The Hitchhiker’s Guide to the Galaxy, in which the handy Babel fish serves as an instant translator when inserted into the ear.
In recent years, there has been growing anticipation that this dream of tearing down the language barrier might become reality. The hope is being driven particularly by artificial intelligence (AI) and its potential as demonstrated in other fields.
Thinking machines
AI refers to the simulation of human intelligence in computers, whereby the machine supposedly acquires the ability to “think” like a human, enabling it to mimic human actions. Examples of applied AI include self-driving cars, facial recognition systems and smart personal assistants, such as Apple Inc.’s Siri and Google LLC’s Alexa.
Further enhancing AI is the concept of deep learning. This refers to how an AI system essentially refines its output and performance through trial and error, an exhaustive process of ploughing through a massive data set of sample inputs.
The effectiveness of deep-learning systems is closely tied to the amount of data used. Meaningful improvement in system performance often requires an exponential increase in the size of the data set.
Found in translation
In the case of machine translation, this deep-learning approach involves an AI-driven system churning through a massive database that contains matched pairs of words, phrases and sentences in two languages. Using these matched pairs, the AI-powered machine translation system identifies frequently occurring patterns within the bilingual data and applies what it has gleaned to each new source document that it is fed. This is how it generates a translation of the document from the source language to the target language.
The computer itself will not have generated these matched sentence-fragment pairs. Instead, the firm manufacturing the system will have already sourced them. For this reason, AI firms will often contact translation firms and ask for access to their bilingual indexes, which will have been produced by human translators. However, this practice may raise ethical questions relating to data protection and client confidentiality.
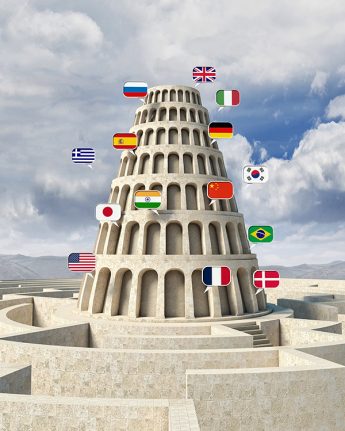
babel tower concept 3d rendering
Fake it ’til you make it
Thanks to the prodigious processing power of today’s computers, machine translation systems are now able to crank out high-volume translations with impressive speed—another factor that endears them to end-users.
It is worth noting, however, that the machine is not comprehending the text in any real sense. Instead, it is simply using the fruits of its extensive analysis of corresponding language patterns in the two languages to create an equivalent text that is as convincing as possible. In other words, the computer is just mimicking the human translator using incredibly extensive observation.
An experienced and knowledgeable human translator, on the other hand, possesses a clear mental picture and an overall understanding of the source text’s subject matter—be it the functioning of a jet engine, a complex legal or foreign-policy issue, the inner workings of a digital camera, or the characteristics of a particular financial market.
The human translator understands the implications of what is written in the source text as well as what is explicitly stated. This enables them to select just the right word, term or phrase, to spot nuance, and to deduce the correct meaning based on each statement’s context. These skills are simply beyond the present capability of computers. Despite all the fanfare surrounding AI, experts in the field quietly acknowledge that solving the problem of natural-language understanding would be the equivalent of solving the problem of human intelligence itself.
For now, some vendors of AI translation systems are making their wares more marketable by having human editors review, edit and clean up the raw output of the machine translation system. This halfway-house solution may well satisfy their clients, who will probably be none-the-wiser as to why their AI-generated translations read as smoothly as the writing of a human translator.
However, this approach would seem to underscore the shortcomings of these systems as much as their potential power. It has also been said that when an AI fails it does not fail gracefully. An example is the self-driving car that drove into the side of a truck which happened to be the same colour as the open road that the car’s imaging systems were attempting to identify.
Similarly, AI translation systems do not appear to have the self-regulating capability necessary to avoid catastrophic linguistic slip-ups, which may have serious adverse consequences for the consumer if they are not detected in time by human intervention.